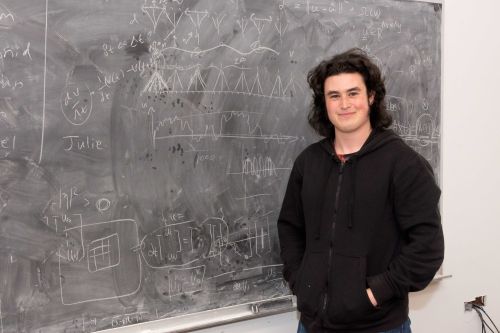
For most people, turbulence is what causes bumpy airplane rides. Turbulence makes the captain turn on the fasten seatbelt sign. Turbulence stops you from being free to move about the cabin.
But Sam Otto will tell you that turbulence is hardly confined to airplanes—and that it’s one of the most common (and complex) pattern-forming behaviors in nature.
“Turbulence is what happens when energy is injected into a fluid, and fluid flows are everywhere,” Sam explains. “If you stir milk into a cup of coffee, you’re creating turbulence. When stars cluster in a galaxy, there’s turbulence. Turbulence can be found in the atmosphere, in the oceans, and in streams.”
Turbulence remains one of the most challenging behaviors to understand and predict, in part because accurately modeling turbulence means accurately modeling each and every wiggle in the fluid’s motion.
“You have to keep track of many variables as they change over time,” explains Sam. “In order to speed up our simulations and advance our understanding, we need to develop a large-scale picture of turbulence that reduces the number of variables involved while still capturing the essential features resulting from small-scale motion in the fluid.”
Turbulent fluid flows contain vortices whose wiggly motions take place over a wide range of sizes or “length scales.” Big motions are unstable and break down into smaller motions through a cascade that eventually dissipates the kinetic energy as heat. Sam wants to extend current modeling techniques by using machine learning to identify good variables for modeling the small length scales and their interactions with the larger scales statistically.
Sam’s Path to Modeling Turbulence Started With Model Rockets
Today, Sam spends most of his time grounding himself in the literature. He reads about artificial neural networks. He reads about the most cutting edge approaches for modeling turbulence—which is something that he started before beginning his PhD program.
“Before Sam enrolled at Princeton, he came to visit.” explains Professor Clancy Rowley. “Even before he arrived, he seemed to have read all of my papers. I was amazed by how much he knew and how solid his foundation was. Plus, he was excited about everything and overflowing with enthusiasm.”
But before he was spending his days immersed in rigorous theory, Sam was a youngster with a model rocket.
“My dad brought home a model rocket kit when I was 5 or 6 years old and we built it together,” explains Sam. “I had tons of questions about how the fins would affect the rocket’s flight. The more questions I had, the more I learned that the only way to answer them would be to study math and physics.”
Before he even began his undergraduate years at Purdue University, he did exactly that. In addition to coursework in fluid dynamics, Sam completed one major research project in gas dynamics. Then he worked on another on combustion in hybrid rocket engines. These projects led to an internship developing large eddy simulations during his freshman year at Purdue. While there, Sam came to a realization that would shape the course of his work.
“At my internship, I learned that turbulence simulations take days to run,” he explains. “If someone wants to see the effect of turbulence on something they’re designing, they need to add days to their design timeline. I realized that simplifying models to run simulations in a few hours or minutes could accelerate the design process.”
The potential commercial impact of Sam’s work is significant. Today, engineers who are designing and optimizing airplane wings need to allocate hours, days, or even weeks to run turbulence simulations. The process is computationally expensive. The design process is slow.
But if Sam can develop a way to accelerate the process of running these simulations, engineers would be able to spend significantly less time waiting and more time designing. Of course, Sam is quick to point out that commercially viable simulation software isn’t likely to appear overnight.
“I’m at the start of what may be a lifetime project,” he explains. “It’s reasonable that I could have something that works well on a toy model and some simple turbulence examples in the next few years. But what I’m hoping for is to devise a general framework that someone else can turn into software. But first, we need to establish the theory.”
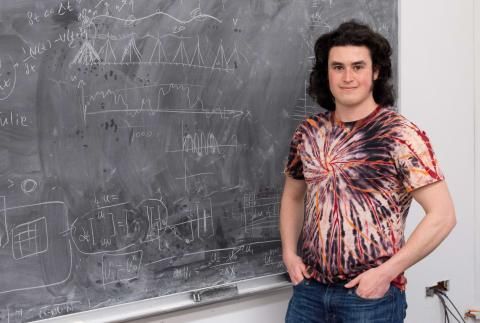
An Interdisciplinary Approach to Modeling Turbulence
Sam’s approach to solving this problem involves an interdisciplinary approach, which is part of why he was excited to begin a PhD program at Princeton.
“The second that I visited I realized that there’s a lot of intellectual freedom, cross-pollination, and support for interdisciplinary scholarship,” he recalls. “What makes Princeton special is that every other grad student you talk to is a true expert in their field. The discussions I’ve had have transformed my own ideas about my research.”
Sam has completed coursework on machine learning with Professor Peter Ramadge and on artificial neural networks with Sy Kung. He’s also working closely with his advisor Professor Rowley, an expert in model reduction for high dimensional systems and fluid dynamics. Together they’re breaking down the complexity of modeling turbulence into simple parts.
“It is a true joy to work with a student who is as enthusiastic, knowledgeable, and creative as Sam,” says Professor Rowley. “He synthesizes new ideas, follows up with calculations, and implements what he’s learned. In one of his most recent papers, he used a neural network to transform a non-linear system into a linear system, which is a clever way of making it easier to understand complicated equations.”
As much of an impact Sam’s work is likely to have on modeling turbulence, his ultimate hope is that it will shed light on other complex problems and challenges.
“If you can figure out a way to simulate turbulence with machine learning, you can simulate many other behaviors,” Sam explains. “Ultimately what we’re doing is using machine learning for pattern identification—and if we start with one of the most complex pattern forming behaviors in nature, we’ll be able to apply what we’ve learned to other patterns. One day we might produce a simulation of galactic turbulence that might reveal something about how they’re formed.”
~Alli Blum