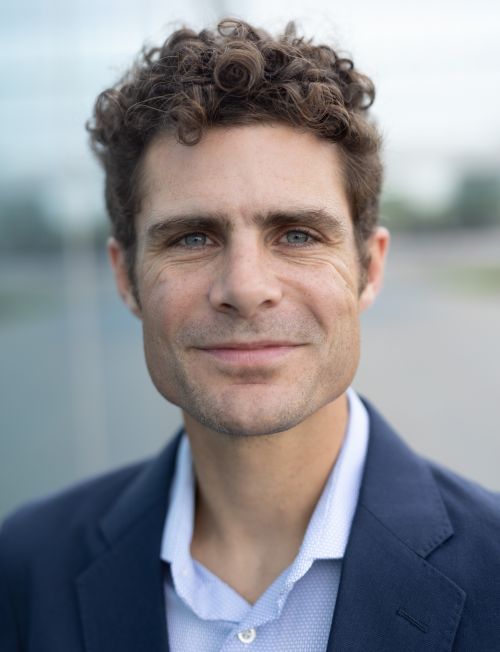
The airfield was Ryne Beeson’s favorite playground growing up. With a father and grandfather who were both pilots, he had the unique opportunity to substitute toy planes and helicopters for real ones.
“What motivated me the most to pursue a career in engineering were all the aviation books my family had laying around,” he says. “I would study the cutaway drawings so I could see how the aircrafts were built.”
Today, as an Assistant Professor of MAE at Princeton, his goal is to make spaceflight design easier. Professor Beeson’s work focuses on “self-optimization”— getting spacecraft from one place to another in the least amount of time, while using as little fuel as possible, and doing so with little human oversight.
As advancements in technology make it easier and cheaper to launch satellites and spacecraft, Professor Beeson is trying to break down the barriers that remain in mission planning and operations. “The workload for humans in this area is actually increasing,” he explains, “and it is creating a bottleneck.”
The solution, Professor Beeson says, is automated mission planning. By leveraging the dynamical structures due to gravitational bodies in the solar system, programs can be designed that find ideal spacecraft trajectories to final destinations without human intervention. For example, a telescope in Earth orbit can use very little thrust to follow a specific spatial path to reach a point between the Sun and Earth, where it will stay mostly stationary.
In the lab, Professor Beeson is developing probabilistic global optimization algorithms that identify and use these dynamical structures. “Math provides us with the ability for different views of complex problems,” he explains. These algorithms have applications both here and far away—for small satellites using electric propulsion and deep space exploration, such as future missions to Jupiter that will need to navigate the complex gravitational environment created by the planet’s moons.
Professor Beeson also brings research in estimation to Princeton. At the University of Illinois at Urbana-Champaign, his dissertation focused on nonlinear filtering theory for high-dimensional systems with applications towards improving estimation in problems related to weather or climate. As a former collegiate cross-country and track runner, he will also work in an advisory role for the academic-athletic fellow program and teach courses in optimal control, space system design, and estimation theory.