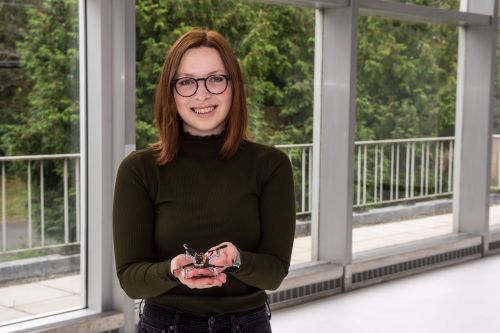
November 1, 2021
By Carolyn Sayre
How much memory does a robot need to complete a task? Are there fundamental tradeoffs between memory and task performance? These are the questions Meghan Booker, a fourth-year PhD student in Mechanical & Aerospace Engineering (MAE) at Princeton, is trying to understand.
Consider a robot that has been assigned a simple task: navigate through obstacles to get to a goal location in the corner of a room. A common solution in the field of robotics has been to give the robot a map. But that solution can be problematic — these maps take up a good deal of memory space, contain irrelevant geometric details about the room and obstacles, and are not a realistic representation of what happens out in the field with uncertain conditions.
“The map is not the memory representation the robot really needs to complete this navigation task. Instead, we can jointly design the control policy and memory representation to follow the wall of the room rather than use the map to navigate through the obstacles. The wall-following policy only uses depth sensor measurements about the wall’s location in memory,” explains Meghan. “Additionally, by relying on the map, the robot ends up taking actions using extra information that might not even be relevant to the task at hand such as colors of the obstacles.”
As part of Professor Anirudha Majumdar's Intelligent Robot Motion (IRoM) Lab, Meghan is trying to automatically generate the minimal amount of memory a robot needs to complete a given task. She is developing memory frameworks that take up minimal amounts of space, while being task-relevant and computationally efficient.
“There is also a theoretical importance to understanding memory for robot tasks and that can help us design better, more efficient algorithms and robotic systems,” she says. “When we enter the real world with all these uncertainties it becomes even more important to have a clear-cut theoretical framework to guide our expectations on what capabilities robots need for a specific task. Right now, it is very ad-hoc.”
Meghan is also investigating memory frameworks that exhibit strong recall so that a robot can complete complex, long term tasks. Examples of important applications for her work are autonomous cars, which handle an immense amount of data, drones, which are smaller and have limited space for memory, and in-home assistance robots, which are expected to handle many tasks over a long time period. These insights into memory, she says, also have applications in other fields such as neuroscience.
“Meghan was one of the first students to join my research group when I started as faculty at Princeton. Her work has helped shape and crystallize our group's efforts. She has contributed greatly to the growth of the research group, both in terms of research and other efforts including student recruitment, outreach, and mentoring undergraduate students,” says Anirudha Majumdar, PhD, Assistant Professor of MAE at Princeton.
Meghan’s interest in engineering began in high school while she was living in New Hampshire. During Project Lead the Way courses, she developed a passion for problem solving and working as a team to tackle STEM challenges such as developing a mini, automated recycling center with VEX robotics and marbles. She was first introduced to robotics during an intensive summer program in artificial intelligence at St. Paul’s School in Concord, NH.
“What I enjoy most about robotics is that it has a feedback loop,” she explains. “Robotics is a constant cycle of developing your hypothesis, making algorithms to actually run on the robot, and then testing your theory and algorithm on a physical robot in the real world.”
Her expertise in electrical engineering parlays well with the control aspects of robotics. As an undergraduate at the Ohio State University (OSU), she majored in electrical engineering and had several internships at Raytheon Company. Meghan’s undergraduate thesis investigated the impact of cyber-attacks on unmanned aerial vehicles connected to the cloud. This work led to Meghan placing in her respective category at OSU’s Denman Undergraduate Research Forum and receiving First Place Paper at the Institute of Electrical and Electronics Engineers Region 2 Student Activities Conference.
At Princeton, Meghan also organizes the Robotics Project Group meetings. This collaborative group of engineers from various departments including MAE, ECE, and COS works together to tackle a unifying task: a team of ground vehicle robots cleaning up trash in environments such as public parks, parking lots after events, or beaches after storms.
As a first-generation college student in her family, Meghan is passionate about mentoring undergraduates at Princeton. She serves as a teaching assistant for Introduction to Robotics and Introduction to Engineering Dynamics. In 2021, she won the Crocco Award for Teaching Excellence in MAE.
“I enjoy working with students to help them find the right direction, whether it be solving a challenging problem or finding a career path,” she says. “I am very grateful for all of the great mentors I had that helped me learn about the opportunities that were out there. Teaching is my way of thanking them and giving back.”