Event Date/Time
Location
Atrium 222
Series/Event Type
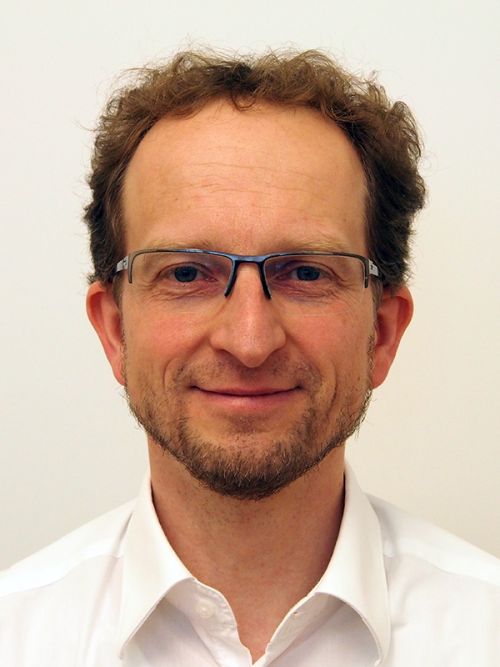
We consider state estimation in discrete-time nonlinear dynamic stochastic systems from noisy measurements of the system output. For representing the desired state, deterministic particles (low-discrepancy samples) are used, where the particles are obtained by minimizing a novel distance measure from a given continuous density. In order to avoid particle degeneration, the filter step is reformulated as a particle flow over an artificial time by progressively introducing the likelihood. Resampling, i.e., the replacement of non-equally weighted samples by equally weighted ones, is performed continuously over the particle flow based on the novel distance measure. Several examples demonstrate the performance of the progressive particle filtering approach.