Event Date/Time
Location
Series/Event Type
Clouds remain one of the greatest sources of uncertainty in predicting future climate, as they involve complex, non-linear processes that extend from the submicron scale to the kilometer scale. Our current ability to model clouds is limited by significant uncertainties, particularly in the intricate microphysical processes that govern the interaction and growth of cloud droplets and ice crystals, as well as in accurately modeling clouds across the relevant temporal and spatial scales for climate. These same uncertainties limit our ability to assess proposed solar radiation management strategies to mitigate climate change.
Recent advances in scientific machine learning offer promising methods to address these challenges. I will discuss several recent studies applying these methods to cloud processes. First, I will discuss how hybrid-physics machine learning models can be used to reduce structural uncertainty in models of ice growth in the atmosphere using in situ observations from laboratory experiments and airborne field campaigns. Second, I will discuss how data-driven reduced order modeling can be used to develop simplified (bulk) microphysics schemes in an unsupervised manner from more detailed microphysical models. Finally, I will discuss how these methods can be used to learn relevant information from high resolution global storm resolving models, to represent cloud processes at the spatial scales needed to accurately predict processes at the climate scale.
Speaker Bio
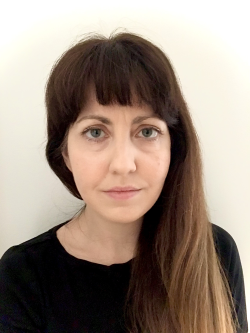