Event Date/Time
Location
Series/Event Type
Despite significant recent advances in robot learning and perception, achieving robust robot behavior for real-world, dynamic tasks like dexterous manipulation remains elusive. This challenge stems from the uncertainty inherent in robots' geometric models, perception systems, and controllers, particularly during dynamic interactions with the environment. This talk explores how risk-sensitivity can provide a principled, practical approach to addressing these robustness issues directly. First, we will discuss our work showing that Neural Radiance Fields (NeRFs) — typically trained for novel view synthesis — can be used for both collision avoidance and localization, repurposing them as a versatile, probabilistic occupancy model for robotics. Next, we will turn to the problem of real-time, risk-sensitive planning more broadly. Specifically, we propose combining stochastic control barrier functions (CBFs), which provide rigorous probabilistic safety/performance guarantees, with deep generative dynamics models to yield a lightweight, data-driven approach to risk-sensitive control. We demonstrate that our method (running onboard a quadrotor at 100Hz) enables aggressive, yet safe flight with a completely unmodeled and uninstrumented slung load. The talk will conclude with a discussion of some lessons learned and future directions in risk-sensitive robotics.
Speaker Bio
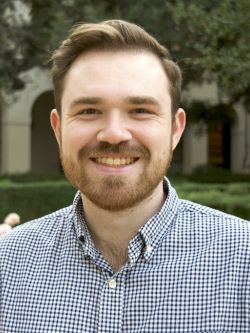